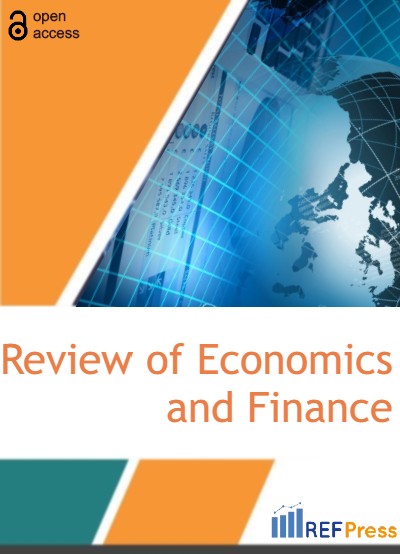
Forecasting Crude Oil Price with Hybrid Approaches
(Pages 564-576)Amina Ladhari Zahouani1 and Heni Boubaker1,2,*
1Institute of High Commercial Studies (IHEC) of Sousse, LaREMFiQ, B.P. 40, 4054 Sousse, Tunisia.
2IPAG Business School, IPAG LAB, 184 Boulevard Saint-Germain, 75006 Paris, France.
DOI: https://doi.org/10.55365/1923.x2023.21.58
Abstract:
The objective of this paper is to study the oil price forecasting using parsimonious models that provide the best possible match using the fewest variables or parameters and to compare the performance of our hybrid forecasting models, namely Artificial Neural Network (ANN)-Long Short-Term Memory (LSTM), Convolutional Neural Network (CNN)-LSTM, Bidirectional Recurrent Neural Network (BRNN)-LSTM, and LSTM-Attention, in predicting the daily oil price. This study uses time series data of crude oil price. The data was preprocessed and divided into training and testing sets. The four hybrid models were developed and trained on the training set. The performance of the models is evaluated by using three metrics: Mean Squared Error (MSE), Mean Absolute Error (MAE), and Mean Absolute Percentage Error (MAPE). The results show that the LSTM-Attention model outperforms the other models in terms of all three-evaluation metrics, with the lowest error rate. Nevertheless, this approach produces smaller predicting errors than the other computing techniques.
Keywords:
Forecast performance, Neural-Networks, Hybrid models, LSTM-Attention, Oil price.
How to Cite:
Amina Ladhari Zahouani and Heni Boubaker. Forecasting Crude Oil Price with Hybrid Approaches. [ref]: vol.21.2023. available at: https://refpress.org/ref-vol21-a58/
Licensee REF Press
This is an open access article licensed under the terms of the Creative Commons Attribution Non-Commercial License
(http://creativecommons.org/licenses/by-nc/3.0/) which permits unrestricted, non-commercial use, distribution and reproduction in
any medium, provided the work is properly cited.