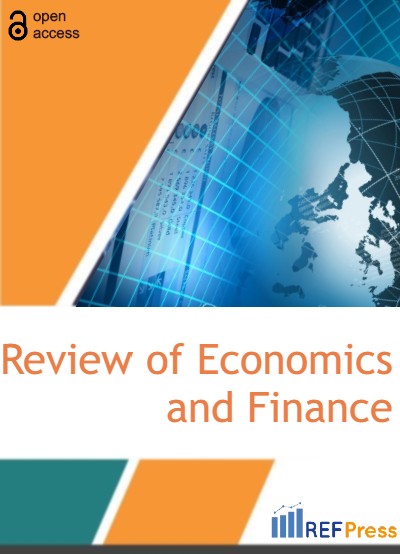
Asset allocation by Unsupervised Learning
(Pages 338-346)Ismail Lotfi1,2,*, Lamiae Megzari3,2 and Abdelhamid EL Bouhadi1,3
1Laboratoire de Recherche et d'Etudes en Management, Entrepreneuriat et Finance (LAREMEF), ENCGF,
Sidi Mohamed Ben Abdellah University, Fez, Morocco.
2Laboratoire Systèmes et Environnements Durables (LSED), Faculté des Sciences de l’Ingénieur, Université
Privée de Fès, Fez, Morocco.
3Laboratoire d’Etudes et Recherche en Management des Organisations et des Territoires (ERMOT), FSJES,
Sidi Mohamed Ben Abdellah University, Fez, Morocco
DOI: https://doi.org/10.55365/1923.x2021.19.34
Abstract:
Modern portfolio theory is closely linked to the concept of diversification. As a result, the most important decision of investor is to make his allocation asset portfolio more and more efficient. Thus, for a given level of risk, the investor seeks to maximise the expected return and minimise the risk by constructing an optimal portfolio. In this paper, we seek to know how unsupervised learning can be used to define the asset allocation strategy. In this sense, we have carried out a comparative study between a so-called classical portfolio, which is based on the modern portfolio theory (i.e. a portfolio constructed on the basis of numerical optimisation) and a portfolio based on unsupervised learning. The aim of this comparison is to look for the best performing method that can give the best asset allocation. Our findings show that the optimal strategy for an ambitious investor lies to the unsupervised learning algorithms that allow a dynamic analysis of portfolio. However, the optimal strategy for a risk-averse investor is still the numerical optimisation approach.
Keywords:
Independent Component Analysis, Efficient Frontier, Asset Allocation, Optimisation, Dynamic Simulation.
How to Cite:
Ismail Lotfi, Lamiae Megzari and Abdelhamid EL Bouhadi. Asset allocation by Unsupervised Learning. [ref]: vol.19.2021. available at: https://refpress.org/ref-vol19-a34/
Licensee REF Press This is an open access article licensed under the terms of the Creative Commons Attribution Non-Commercial License (http://creativecommons.org/licenses/by-nc/3.0/) which permits unrestricted, non-commercial use, distribution and reproduction in any medium, provided the work is properly cited.