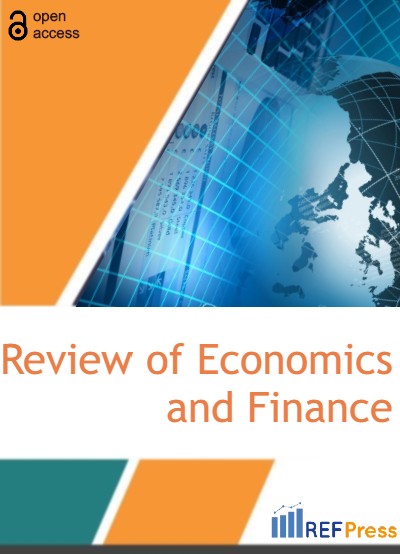
Improving Global Stock Market Prediction with XGBoost and LightGBM Machine Learning Models
(Pages 2603-2610)Omaima Guennioui*, Dalila Chiadmi, Mustapha Amghar
Mohammadia School of Engineering, Mohammed V University in Rabat, Rabat, Morocco.
DOI: https://doi.org/10.55365/1923.x2023.21.278
Abstract:
Predicting stock market prices has been a challenging task for analysts and researchers over the years due to the numerous factors that affect stock prices, making it difficult to achieve accurate predictions. This paper focuses on the application of machine learning techniques, specifically Light Gradient-Boosting Machine (LightGBM) and Extreme Gradient Boosting (XGBoost), to forecast stock market trends. Furthermore, it introduces a hybrid model called Global XGBoost-LightGBM, which combines these individual models. The study adopts a global approach by consistently applying parameter settings across multiple series and evaluates the models using a significant number of stocks. The effectiveness of this approach is validated through experimentation in two distinct stock markets: US and Morocco. Experimental results clearly demonstrate that the integration of LightGBM and XGBoost enhances the ability to identify and interpret fluctuations in stock prices, enabling these models to effectively address the challenges associated with stock market prediction.
Keywords:
Global Forecasting Models, Machine learning, Regression, Stock forecasting.
How to Cite:
Omaima Guennioui, Dalila Chiadmi, Mustapha Amghar. Improving Global Stock Market Prediction with XGBoost and LightGBM Machine Learning Models. [ref]: vol.21.2023. available at: https://refpress.org/ref-vol21-a278/
Licensee REF Press This is an open access article licensed under the terms of the Creative Commons Attribution Non-Commercial License (http://creativecommons.org/licenses/by-nc/3.0/) which permits unrestricted, non-commercial use, distribution and reproduction in any medium, provided the work is properly cited.