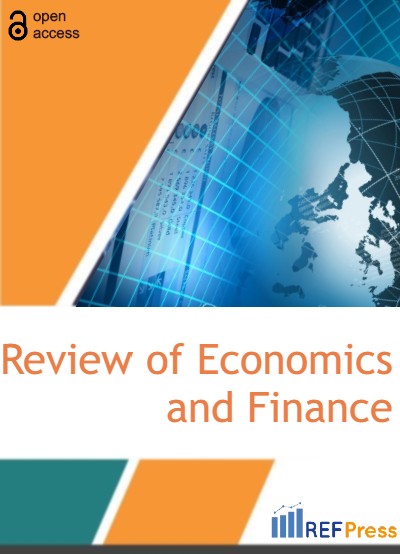
Liquidity Classification of Equities Under Stress Using Machine Learning Models: Evidence from Major World Share Indices
(Pages 1317-1331)Coskun Tarkocin1,* and Murat Donduran2
1phD Candidate in Economics, Graduate School of Social Sciences, Yildiz Technical University, Istanbul, Turkey.
2Director of Graduate School of Social Sciences, Yildiz Technical University, Istanbul, Turkey.
DOI: https://doi.org/10.55365/1923.x2023.21.144
Abstract:
The classification of assets based on their liquidity behaviour under stress is a crucial element of bank liquidity stress testing. It is also important to define how financial institutions should fund these assets within the current business model whilst avoiding excessive liquidity risk. This study aims to revisit the liquidity coverage ratio (LCR) assumptions for common equity shares using new data attributes and supervised machine learning models. This research contributes to the literature by providing fresh insight into which characteristics impact share behaviour under liquidity stress. Empirical results suggest sector, share beta, industry, and market capitalisation of the share are contributing factors which help predict shares’ liquidity behaviour under stress. This study also finds that the financial, basic materials and energy sectors are more volatile and less liquid under market stress; shares with lower beta show more liquid characteristics, and higher market cap stocks show more liquid behaviour.
Keywords:
Liquidity Risk, Equities, Liquidity Coverage Ratio (LCR), Machine Learning Models, Ensemble Model, Random Undersampling Algorithm, financial stress.
JEL Classification:
C10, G01, G21, G33.
How to Cite:
Coskun Tarkocin and Murat Donduran. Liquidity Classification of Equities Under Stress Using Machine Learning Models: Evidence from Major World Share Indices. [ref]: vol.21.2023. available at: https://refpress.org/ref-vol21-a144/
Licensee REF Press This is an open access article licensed under the terms of the Creative Commons Attribution Non-Commercial License (http://creativecommons.org/licenses/by-nc/3.0/) which permits unrestricted, non-commercial use, distribution and reproduction in any medium, provided the work is properly cited.