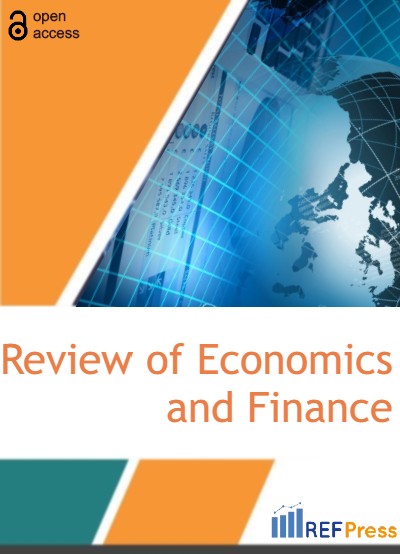
Forecasting US Output Growth with Large Information Sets
(Pages 13-16)Afees A. Salisu1, Umar Bida Ndako2 and Rangan Gupta3,*
1Centre for Econometric & Allied Research, University of Ibadan, Ibadan, Nigeria.
2Economic Policy Directorate, Central Bank of Nigeria.
3Economics Department, University of Pretoria, South Africa.
DOI: https://doi.org/10.55365/1923.x2021.19.02
Abstract:
We forecast US output growth using an array of both Classical and Bayesian models including the recently developed Dynamic Variable Selection prior with Variational Bayes [DVSVB] of Koop and Korobilis (2020). We accommodate over 300 predictors that are incrementally captured from 5 factors, 60 factors to over 300 factors covering relevant economic agents. For robustness, we allow for both constant and time varying coefficients as well as alternative proxies for output growth. Using data covering 1960:Q1 to 2018:Q4, our results consistently support the use of high-dimensional models when forecasting US output growth regardless of the choice of forecast measure. For the density forecast of real GDP growth in particular, the results favour the DVSVB and time varying parameter assumption.
Keywords:
US Output Growth; High-Dimensional Models; Forecast Evaluation.
JEL Classification:
O41, O51, C52, C51, C53.
How to Cite:
Afees A. Salisu, Umar Bida Ndako and Rangan Gupta. Forecasting US Output Growth with Large Information Sets. [ref]: vol.19.2021. available at: https://refpress.org/ref-vol19-a2/
Licensee REF Press This is an open access article licensed under the terms of the Creative Commons Attribution Non-Commercial License (http://creativecommons.org/licenses/by-nc/3.0/) which permits unrestricted, non-commercial use, distribution and reproduction in any medium, provided the work is properly cited.